In earlier articles in this series, we have discussed the various functions within any business that can contribute to sound equipment reliability (design, operations, maintenance and supply). We have also looked at the key tools and techniques that can be used by these functions, and where these may be applicable.
In this article, we will continue our exploration of reliability topics by considering how developments such as the Industrial Internet of Things (IIOT), big data and analytics are contributing to reliability improvement.
We have previously explored the impact of these development on maintenance in our article Big Data, Predictive Analytics and Maintenance. We have also explored the impact of these developments on traditional thinking about predictive maintenance in our article The PF Interval – Is it Relevant in the world of Big Data?
In this article, we will extend this exploration into the world of reliability engineering. Specifically, we will consider how the abundance of accessible and consistent data, combined with applications to support visualisation, modelling, analysis and machine learning are supporting the four key pillars of modern reliability engineering, namely:
- Reliability by Design,
- Operate for Reliability,
- Maintenance Tactics Optimisation, and
- Defect Elimination
This is the seventh article in a series of articles on Reliability Improvement. The other articles in this series include:
Articles in this series
In our next article, we will explore the challenges of ensuring that reliability improvement processes, and specific reliability improvements are sustainable and the roles that leadership, culture and appropriate metrics play in driving this sustainability.
Big data, predictive analytics and maintenance
Much of the conversation around IIOT, Big Data and Predictive Analytics has been in relation to the potential these developments offer to more timely and targeted maintenance. Most of today’s literature focusses on the progression from diagnostics to prognostics to prescriptive maintenance.
In the diagnostics domain, businesses are making use of new sensor and analytics technology to enhance their condition monitoring programs. They are using these technologies to predict imminent failure earlier so that they can better plan maintenance, or using improved technologies to more closely track failure progression and fine tune the timing of a maintenance intervention. We describe this in our article The PF Interval – Is it Relevant in the world of Big Data?.
In the prognostics domain, real time sensor data is combined with understanding of overall asset condition and failure characteristics to not only detect an imminent failure, but also to make a determination of remaining life.
The prescriptive domain is the ‘ultimate’ aim for today. Here we combine understanding of the failure and condition of the asset with an understanding of consequences of failure to determine what to do and when to do it. At its simplest, this could be to trigger a fully populated work order for a maintenance intervention. However, the prescriptive domain can also mean so much more. Imagine being able to trade off failure risk, production options, maintenance costs on a real time basis to make the best business decision about whether to intervene, when to intervene and what action to take and to continually refine this decision as the failure progresses.
As exciting as these advances seem, the focus on optimising maintenance decisions is only half the puzzle. The other half is how these emerging technologies and application can support the elimination of failures in the first place.
Changing the game for reliability
So, what is it that the IIOT, Big Data and Analytics brings to the table to enhance equipment reliability?
In our earlier article ‘Big Data, Predictive Analytics and Maintenance’ we described the Internet of Things as the network of physical objects or “things” embedded with electronics, software, sensors, and network connectivity, which enables these objects to collect and exchange data.
We then described Big Data as the large amounts of data that are available from disparate systems (such as ERP systems, process control systems, condition monitoring systems etc), and more importantly advances in information technology that now make it possible to store and analyse a more complete picture of our assets, based on a complete set of data drawn from a variety of sources.
The advances in the way we manage data and technologies that enable us to store and analyse this data to improve maintenance also enable us to enhance reliability.
Data
Advancing technologies have changed the way data can be used to add value to the business:
- Open Standards – A key advance has been the move to more open data standards. In the past, sensors have been connected via proprietary communications protocols, and their outputs have usually only been available for viewing via a proprietary control interfaces. These devices can now communicate using standard internet communications protocols, using open standards, and as a result, the data is now available for a broad range of uses.
- Commonality – In the past, challenges around common naming and numbering conventions often limited our ability to connect the dots between data from disparate systems. Today’s technologies are breaking down these barriers, even enabling unstructured data such as text comments to be connected to structured data to support analytical capabilities that were not thinkable a decade ago.
- Persistence – Advances in data storage have seen us progress from PLCs storing perhaps 24 hours of data, to plant historians that could store weeks or months of data, to ‘data lakes’ that have ever expanding shorelines. And with this longer history of higher resolution data come the ability to perform more detailed analysis.
- Accessibility – the removal of barriers around data structures and communication protocols combined with advances in data storage means that data can now be more readily shared between functions within an organisation, and between organisations – for example, equipment manufacturer and customer.
Applications
The applications available to make use of data have also improved exponentially. Reports and dashboards that were once the domain of IT departments are now within reach for most users via advances in visualisation tools. Business and process models can now be informed by real time data to self-adapt and learn, and better represent the real world. And powerful analytical tools that were once the domain of supercomputers and a small community of specialists are now available to businesses as server and even desktop applications able to be used by the ever-growing disciple of ‘data scientists’.
The four pillars of reliability
So how do these advances in data and applications impact reliability. Let’s look at the four key pillars: Reliability in Design, Operate for Reliability, Preventive Maintenance Strategies, and Defect Elimination.
Reliability in Design
IIOT and Big Data are already having a significant impact on design. In the past, equipment manufacturers sold equipment, and their most reliable sources of information about in-field performance were warranty claims, spare parts orders and customer complaints. Today many manufacturers are being fed real-time or near-real-time data about their equipment and have much better visibility of machine performance to inform modification of existing equipment and design enhancements for future equipment.
This extends from the humble motor vehicle with its engine management system recording data that is downloaded during servicing, through to large mining equipment beaming telemetry data back to the manufacturer via satellite, to aircraft all over the planet sending live engine telemetry to the manufacturer.
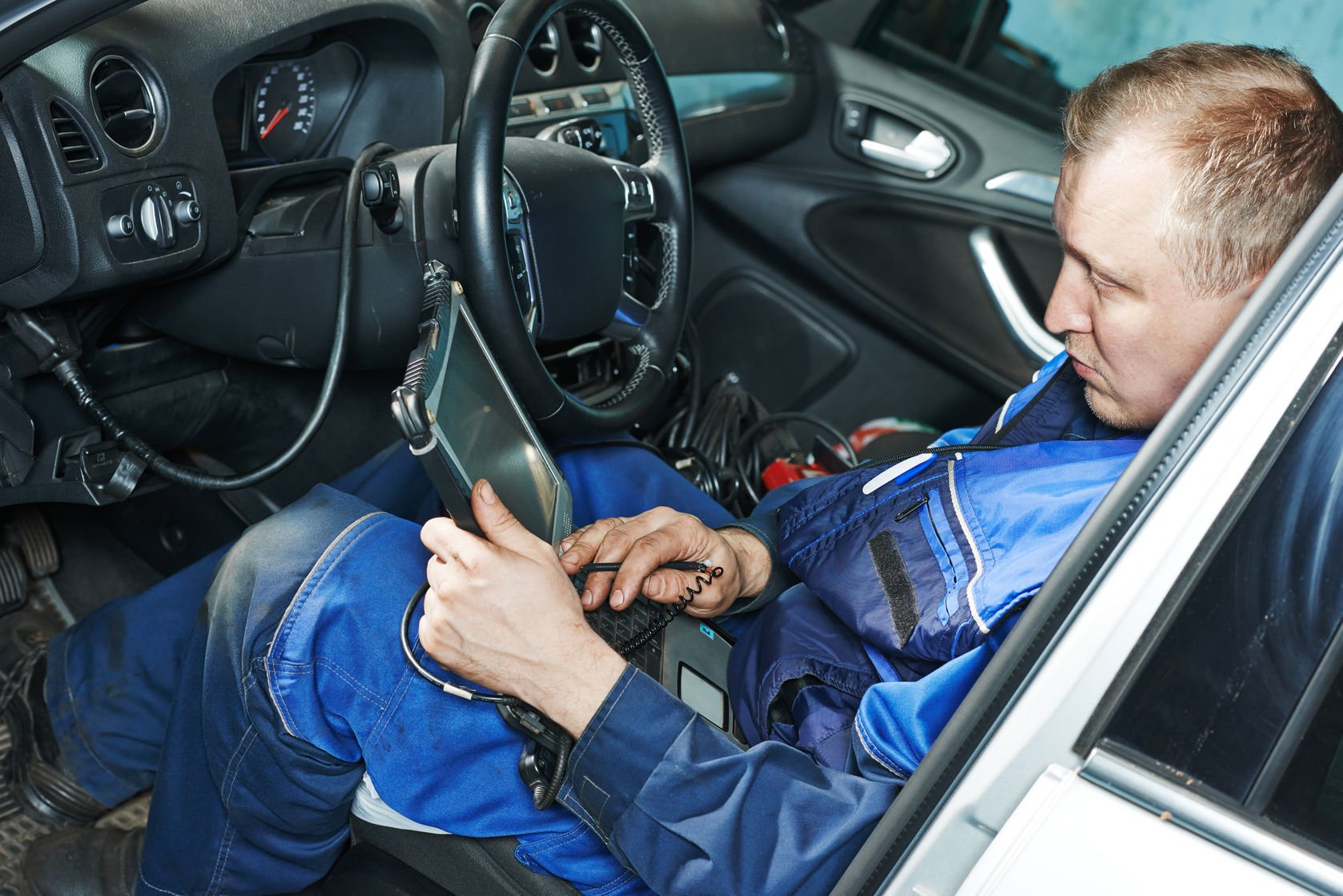
Similarly, within manufacturing and processing facilities, these same technologies can be used to better understand plant performance and improve reliability.
This improvement can be related to individual machine reliability … we will cover this below in Defect Elimination. More importantly, by considering the manufacturing or processing facility as a system, we can focus on maximising system reliability by making real time decisions that match production rates to productive capacity, capitalise on redundancy and alternate pathways, and load share to distribute wear and tear and optimise overall system performance.
Operate for reliability
While it is widely recognised that choosing the right equipment in the first place has the most significant impact on reliability, the next most significant impact is the way that the equipment is operated and ensuring that it is not operated outside of its intended operating envelope. Again, taking the humble motor vehicle, we take for granted sensors that alert the driver to all manner of issues from low oil pressures or high engine temperature, to deflating tyres and failing alternators.
In a similar way, IIOT allows us to better inform human operators when they are about to stray beyond the equipment envelope. Alarms and alert are simple form of this. But this same data can be used to inform training needs to produce better operators rather than detecting events as they occur. Take for example mining haul trucks where gear change events, brake application, speed, load, and engine RPM can be monitored from afar to observe operator behaviours that need to be corrected, and then develop scorecards and training programs accordingly.
The move to an automated world will demand even more of this technology. In the past, as we drove our motor vehicle to work, a squeal from the rear of the car as we turned a corner, combined with an odd ‘feel’ alerted us to a flat tyre. Today, we are just as likely to see a warning light that we have low tyre pressure. But fast forward to the world of an automated vehicle on its way to collect a passenger … it does not have the luxury of a driver to determine the most appropriate intervention. It will rely on a prescriptive algorithm to determine the most appropriate action.
Preventive maintenance strategies
Our earlier article ‘Big Data, Predictive Analytics and Maintenance’ describes how advancing technologies offer new methods of monitoring condition, detecting the onset of failure, and fine tuning an intervention.
Tradition FMECA and PM optimisation approaches have relied on Work Order data to understand parameters such as mean time between failure. Reliability analysts are often challenged by incomplete data … breakdown events that do not result in a work order, events that are recorded against a parent equipment and not counted in the component failure rate etc.
Sensor data collected, stored and available for analysis provide a much more consistent and reliable set of data to support this analysis.
Defect elimination
The final pillar is the Defect Elimination process – proactively identifying sources of loss, prioritising these losses for investigation and resolution, conducting a root cause analysis to identify actions to eliminate the loss, tracking actions, and confirming that the actions actually eliminated the defect.
In the past, loss and delay accounting data has typically been used to identify improvement targets. While acute (one-off, major) losses typically stand out and are easy to identify, chronic (frequent but less severe) losses are sometimes harder to discern – different operators may code the same failure in different ways, etc. Further, while for acute failures the cost of lost production typically outweighs maintenance costs, for chronic failures, maintenance costs can start to stack up and even outweigh production losses.
Using sensor data to better inform the delay and loss accounting system leads to more accurate and consistent capture of delay and loss events, while the ability to blend this data with maintenance cost data using big data tools provides the ability to pinpoint major sources of loss.
This same data can be used to support the root cause analysis process. In some cases, the cause of failure may be evident, but in others, the availability of persistent high-resolution data may offer previously unavailable information on the cause of the failure.
The final step in the Defect Elimination process is the ensure the defect has actually been resolved. Again, the availability of persistent high-resolution data means that assessing performance before and after the resolution has been implemented is readily measurable.
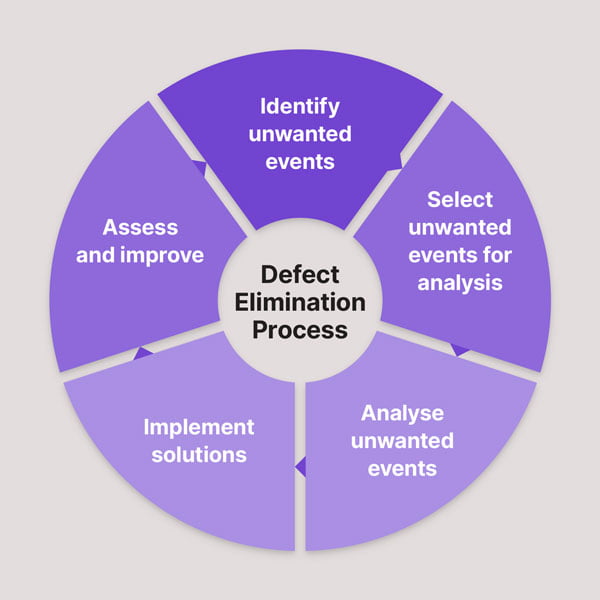
A word of caution
There can be no doubt that the Industrial Internet of Things and Big Data are reshaping how we think about maintenance and reliability. Often the push to capitalise on these technologies is driven by the IT department who are all too happy to invest significant effort and funding to build the ‘data lake’ and then go looking for ‘swimmers’!
We believe that implementing these technologies should be about solving business problems – not about investing in the latest shiny thing for the sake of it, and our aim is to work with clients to determine how emerging technologies can support their business and provide pragmatic, cost-effective and implementable advice.
Conclusion
This article has outlined four tools that can be used to improve equipment reliability. We offer training courses that provide more insights into these (as well as other) reliability improvement tools and methodologies:
-
Product on saleRoot Cause Analysis for Team MembersOriginal price was: $2,150.00.$1,935.00Current price is: $1,935.00.
-
Product on saleReliability Excellence FundamentalsOriginal price was: $4,795.00.$4,315.50Current price is: $4,315.50.
-
Product on saleRCM & PMO for Team MembersOriginal price was: $2,150.00.$1,935.00Current price is: $1,935.00.
-
Product on saleIntroduction to Reliability ImprovementOriginal price was: $1,075.00.$967.50Current price is: $967.50.
If you would like to receive early notification of publication of future articles, sign up for our newsletter now. In the meantime, if you would like assistance in establishing effective reliability in operations within your organisation, please contact me. I would be delighted to assist you.