This is the first in a series of two articles on Condition Monitoring (CM). This series will cover:
- How Condition Monitoring can be used to determine Asset Health;
- The current status of Condition Monitoring in terms of technological development
- What systems and processes can be used to establish and manage a successful Asset Health and Condition Monitoring program.
In this article, I will give you an overview of the current state of the art in this field, a perspective of what a Condition Monitoring system looks like as well as its main components and their interactions.
The current state of condition monitoring
The trend is clear; data collection is now ubiquitous and pervasive. Data is being collected on just about every imaginable variable that can be measured, from the rise of sea level to the number of times you have operated your dishwasher in the last twelve months.
Data is so easy to gather that there are websites that allow users to connect a device to the website and upload live data. The website then allows users to graph and track the measurements. As one example, these types of websites were used by Japanese citizens to setup a network of radiation measuring devices within days of the Fukushima nuclear plant disaster, allowing people to track the spread of the radiation cloud live, as things happened.
Not long ago, I had the opportunity to work with a client who was monitoring more than 20 or 30 variables in each of their underground electric loaders at a rate of several measurements per minute. This included measurements of the loading speed, the force exerted by each hydraulic ram, and many more.
Improvements in the design and manufacture of electronic components, have allowed suppliers to produce measuring instruments that are increasingly smaller in size, higher in processing power and cheaper to buy.
If you are involved in selecting, installing, operating or maintaining equipment, it is more than likely that you have experienced this in one form or another. You would have been approached by at least one equipment vendor offering the latest monitoring add-on with the promise of improving the reliability of your plant. And it makes sense, or does it? Follow me in this logic.
Good maintenance practices indicate that maintenance efforts need to be focused on preventing rather than fixing failures. In order to detect and prevent equipment failures we need data. The more data you have, the more accurate your assessments will be. More data means better predictions, right? Well, it turns out that it’s not that easy.
The first question you’d have to ask is “why do I need Condition Monitoring?” Regardless of what anyone tells you, the solution has to be the right one for your particular strategic goals and operational needs. Like in many other aspects of Asset Management, one size does not fit all.
Before you embark into establishing a program like this you have to be clear on what your goals are and why it makes sense for your operation to implement this particular solution.
The first question that I would recommend to ask yourself when implementing a CM solution is – “what am I trying to solve?”
The condition monitoring cycle
Although it is true that each situation has to be looked at in its own context, environment and circumstances, it is useful to first use some common language and outline a common process so we can understand the various components of a CM program.
The best way to consider Condition Monitoring is as a cycle, which helps us to remember that this is not a linear system with a beginning and an end, but rather a continuous loop of data acquisition, analysis and decision making that ultimately leads to better Asset Management.
To better illustrate this point, have a look at the following figure.
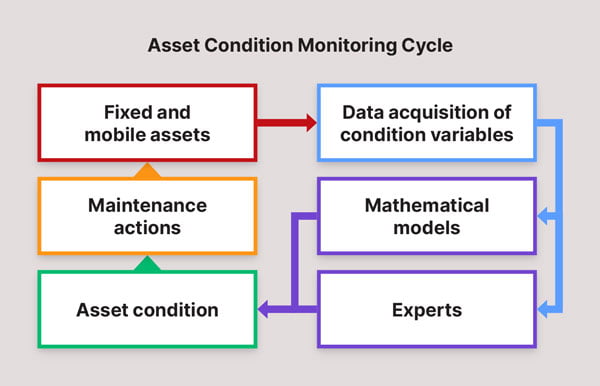
The first block in this cycle is the one that should not be difficult to understand. There are plenty of devices in the market that will measure, store and communicate the variables that you are interested in.
At present, the main challenge is in the part of the process represented with a cloud; the models and experts used to evaluate the data that is being collected and produce a judgement of the status of equipment “health”. In essence, the challenge is how to make sense of the flood of data streams coming in from the devices installed in the field. While we would like to think that the models used to establish the condition of a particular asset are known and predictions are reliable, the reality is that this is not always the case.
Some systems or portions of them will have a simple model and therefore the health diagnosis is relatively easy. These are the types of conditions that can be summarised with “if” rules.
“If temperature [X] is less than set-point [Y] then [asset-condition] is OK, otherwise, raise alarm”.
However a good number of systems are not that simple. They have an intrinsic degree of complexity in the way they operate and respond to the duty and environmental conditions they are subjected to. This is even more evident to anyone that has been asked to evaluate risks and conditions of whole plants, where the health of many of these systems and sub-systems needs to be combined into an overall health indicator or KPI.
There is an expectation that at some point the science of Artificial Intelligence will be developed enough to produce a robust automated model and diagnosis tool, but we are not there yet and we’ll leave that for the topic of a future article.
In the meantime, what you can do is to establish a sensible and well-targeted system that will help you reach your strategic goals in terms of reliability and availability without consuming a lot of your resources.
In part two of this series, we will discuss the steps that you could follow to implement a successful CM program.